Artificial Intelligence
AI in Law Enforcement: Governance and Oversight for a Safer Future
Artificial intelligence (AI) is revolutionizing law enforcement, offering powerful tools for data-driven decision-making and predictive analysis. However, its implementation raises concerns about fairness, citizens’ rights, and data privacy. This article explores the key considerations for integrating AI in policing, including ethical vendor selection, scalable systems, officer training, and public trust. By addressing these issues through responsible governance and oversight, law enforcement agencies can ensure AI technologies are used ethically and effectively, fostering a safer and more transparent future for all.
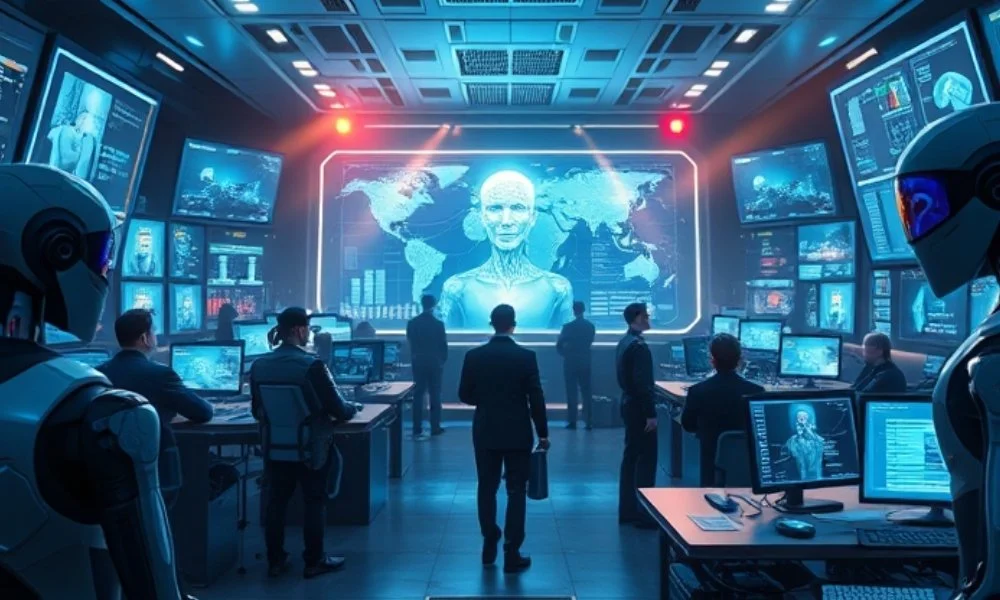
Artificial intelligence as an application in policing is a tool that is changing the way police functions, do their work and enhance the predictive analysis. In spite of this technology having great potential in enhancing human life, the use of this technology comes with various ethical and privacy issues. This paper discusses one of the most contentious issues with concern to the use of AI governance in law enforcement, which is the issue of fairness and citizens’ rights.
Definition:
In this guide, there will be ways to correctly implement artificial intelligence into law enforcement, established on methods of ethical usage, vendor choices, officer training, and compliance with the law. Read on to find out how these vital issues can be solved.
How Can We Evaluate Vendors Effectively?
Selecting the proper vendor is the first approach in developing an acceptable AI strategy in cops. To keep the technology suitable for the goals of law enforcement agencies, every vendor has to meet certain requirements.
Conduct Background Checks:
Check the vendor’s past experience and licenses from previous clients especially from police departments.
Assess Technical Reliability:
Assess the performance of AI algorithms for the stability, fairness and the general reliability.
Ensure Transparency:
Examine how the vendors manage their data sources, training, and constraints of the system.
Use Third-Party Audits:
Ensure that an independent organisation reviews the vendor’s systems for bias and accuracy that is not apparent on the surface.
Negotiate Performance Contracts:
Develop proposed agreements that will have clear delivery provisions, hold bar provisions and demobilization provisions for non-performance.
Verify Compliance with Regulations:
Make sure that the vendor complies with all the applicable laws and regulations of the industry. This also includes data privacy regulations like GDPR or CCPA and any other specific to the industry with which the company is involved in. They also threatened to impose fines or otherwise suffer reputational losses.
Check for Ethical Guidelines:
Determine whether the vendor has an adequate ethical practice to govern the AI systems they develop. Examine their integrity, responsible and responsive policies for fairness, accountability, and transparency and more so, how they mitigate nuisance effects of their AI solutions.
Evaluate Vendor Support:
Assess how much support you should expect from the vendor and what kind of support it is. This includes its availability for identification in case of problems or errors, quick response to inquiries and constant update or support. When support is strong, there are few, if any, hiccups that threaten to disrupt an organization’s performance.
Examine Integration Capabilities:
Check how well these solutions support your current architecture and your organizational processes. Consider how compatible the software is, how simple it is to implement, how easily you can modify it to suit your organization’s framework.
Review Data Management Practices:
Also, require an explanation as to how the vendor approaches the management of data: collection, storage, as well as use. Make sure that the data that is collected and stored is protected in the best way possible and adheres to the privacy act, being used responsibly to prevent any legal or ethical problems.
Analyze Long-Term Viability:
Depending on the vendor, its financial standing, experience, and plans for the future that will demonstrate if the vendor can deliver ongoing support and development. Dealing with a sustainable vendor eliminates the problem of having a technology product that is no longer supported or outdated.
Look for Scalability Options:
See if the solution provided by the AI is likely to grow or expand as your company expands. Availability is an ability of a system to accept more data, more users or become more complex without incurring much cost or resources.
Understand Customization Potential:
Check whether the AI solution has the possibility of a customized solution to fit your needs. A system that is malleable enough to be customized to your needs will give more value and functionality.
Inspect Testing and Validation Procedures:
Request for information from the vendor regarding how they conduct their testing and validation of the developed AI systems. Evaluate the workflow of error identification techniques, elimination of bias, and validation of results before they are used in your organisation.
Evaluate Ethical AI Training Programs:
It also worth to find out if the vendor trains its AI models in ethical behavior. Training should focus on how to reduce bias and how to be more inclusive and ensure system behavior is acceptable in society and achieves organizational objectives.
The effectiveness of AI systems will increase proportionally to the extent that law enforcement teams provide detailed scrutiny to vendors.
Are Our Systems Scalable?
Another important feature is scalability to guarantee that AI solutions extend to cater for the increasing law enforcement problems and growing databases. It also raises the question of how small agencies or an understaffed department can be sure that their systems are scalable?
Compatibility First:
Evaluate whether the use of the AI system is complementary with other databases to support all the departments.
Update IT Infrastructure:
Ensure that your systems can support processing at a massive scale of AI with no interruptions.
Design Modularity:
Purchasing solutions that are flexible, and in some way, component based to adapt to the changing requirements of law enforcement agencies.
Prioritize Interoperability:
Application of standards will encourage the cooperation between agencies and jurisdictions.
Plan Resource Allocation:
Make tools, infrastructure and manpower adaptable in order to leverage the Use of AI.
Assess Cloud Integration:
Assess the position of cloud services in enabling the AI business to achieve scalability, security, and effectiveness when integrating into the existing facilities effectively.
Ensure Data Redundancy:
Always ensure that good and efficient measures of backing up the data as well as replication in the case of system failure.
Optimize Network Bandwidth:
Optimise the network performance to transfer and process huge amount of data with less or no delays.
Evaluate System Load Balancing:
Process and divide tasks with the goal of optimizing server load to avoid overloading and slowing the system down.
Consider Future Technological Advancements:
Ensure that there is a plan for the adoption of emergent technologies by ensuring that up and running systems are flexible and future capable.
Plan for Disaster Recovery:
Closely integrate the best disaster recovery plans that allow organizations to quickly resume operation in case of failures or cyber-incidents.
Monitor Performance Metrics:
We also should systematically assess the performance of system, define which aspects should be optimized to maintain stable productivity.
Implement Automated Scaling Solutions:
Utilise intelligent scaling processes for fine tuning of resources in order to accommodate changing workloads.
Test System Flexibility Regularly:
Practice regular checking to ensure that systems are capable of responding favorably to changes in requirement or design.
Establish Vendor Scalability Support:
Work with vendors that offer solutions that can expand as the agency expands by integrating features pertinent to the agency.
This way you guarantee scalability from the outset and hence the future upgrading and extension will always be a breeze.
What Training Do Teams Need?
Even the most sophisticated AI system can be said to be useless in the absence of training. Ethics, technology and AI limitations training helps the teams to be ready for what they are going to face in the field.
Build AI Literacy:
Sensitize law enforcement officers on what AI IS and is not capable of doing and other right uses of the technology.
Simulate Real Scenarios:
Make people sit through a training, and simulate real-life scenarios for an officer to show him how to implement AI.
Encourage Continuous Learning:
Maintain up-to-date personnel with constantly running courses in the latest AI technologies.
Collaborate with Experts:
Conduct sessions that involve developers, ethicists and community members to ensure skills are well rounded.
Evaluate Success Periodically:
Make sure that training programs are assessed using tests to help in figuring out the efficacy of the training programs.
Integrate Hands-On Workshops:
Spearhead applied sessions that allow participants to have a first-hand experience of the machine intelligence and to complement the theoretical learning.
Promote Ethical Decision-Making:
The ethics of artificial intelligence inclusion should be comprised of special modules to show where bias might exist and how to handle it responsibly throughout all uses of artificial intelligence.
Leverage Cross-Disciplinary Training:
Consult with experts from the fields of psychology, law, data science and others to get more variables, and the potential for the application of AI law enforcement and related risks.
Provide AI System Troubleshooting Skills:
Inform personnel about the possible AI system failures to be able to recognize them and fix in order to avoid time loss.
Implement Scenario-Based Testing:
Arrive at models which present a situation that forces the trainees to apply the acquired knowledge in an innovative manner in view of a real-life situation.
Focus on Data Security Training:
Stress on the protection of critical information within AI structures and also the ways like encryption and access control and response to threats.
Offer Role-Specific AI Training:
Align data with the principal activities of the job and therefore make the training material specific and practical to the position.
Utilize Peer Learning and Mentoring:
Promote knowledge acquisition by supporting the matching of experienced workers with new learners in order to support development of all the employees and the teams.
Incorporate Feedback from Community Stakeholders:
Engage the members of relevant communities in defining the AI training courses that best meet the public needs and fair to all.
Foster Collaborative Problem-Solving Skills:
Case studies that were meant to be solved in groups that are also going to face AI-related issues, which would allow developing the people’s collaboration and creativity.
It has been established that an informed team will benefit from AI while respecting the limits of the technology.
What Does Ethical AI Look Like?
If there are no proper ethical standards in place, then police work has the potential for, and often is, abused and discriminated. What legal standards hold AI to account on how ethical they are?
Establish Core Principles:
Enhance equality, rationality and responsibility as all decisions made should complied with the rights of every citizen.
Focus on Bias Reduction:
Develop ways for detection, prevention and management of bias within the context of AI algorithms.
Invite Community Feedback:
Involve the advocacy groups, the ethics committees and the citizens for audit and further disclosure.
Conduct Ongoing Risk Assessments:
Review operational and societal risk associated with AI implementations to reduce their consequences.
Assign Accountability:
Define, especially at the organizational, group and individual levels, expectations and penalties for non-ethical behaviour.
Promote Fairness and Equity:
Create AI solutions which can decrease the prejudice of the systems they work within, using fairness principles and inclusive data, providing equal opportunities and outcomes for all.
Ensure Data Privacy Protections:
Ensure that the personal data protection is done by strong encryption techniques and anonymization methods and ensure that the laws and regulations of data protection and consent processes are followed.
Foster Transparent Algorithmic Decisions:
Provide an easy to understand explanation of how the algorithms work, what certain assumptions were made and what cannot be achieved with AI decision making systems to the various parties involved.
Develop Ethical AI Governance Policies:
Introduce legal structures that state the ethical approach to AI in law enforcement, make organizational values in this sphere more coherent, and ensure compliance with the same rules across organizations.
Commit to Continuous Ethical Audits:
Schedule annual reviews where the ethical performance of the AI system is evaluated against set benchmarks alongside addressing biases and adverse effects immediately in order to avoid long term implications.
Engage in Regular Public Discourse:
Continuously engage the stakeholders in the society, academia and other professionals who remain relevant in the implementation of AI projects in order to foster their appreciation by constant communication.
Create Ethical AI Use Guidelines:
Develop a set of best practices of artificial intelligence use based on its definition of misuse that could serve as a reference for developers, implementers and end users.
Provide Ethical AI Education and Training:
Implement extensive orientation sessions that provide the teams with information regarding ethical principles in AI, how to identify bias in development, and legal requirements regarding the usage of AI.
Implement Clear Reporting Channels:
Ensure there are common reporting channels where people can report instances of unethical usage of AI or misuse of the technology, so that appropriate action can be taken immediately.
Encourage Ethical AI Collaboration Across Agencies:
Build multi-sector collaborations to share successes, research, and ethical progress, and encourage and promote responsibility-based innovation.
Thus, I want to emphasize the importance of the ethical groundwork as the primary condition for the effectiveness and credibility of AI projects in the police force.
How Can We Build Public Trust?
The proper and successful implementation of an audit requires that the public has trust in the process. Information helps to disarm suspicion and leads to people’s cooperation.
Host Community Meetings:
Host public meetings, especially concerning AI use cases in policing, especially to close knowledge divides.
Maintain Transparency:
Make readily available to the public reports on the use of AI, its procedures, and performance.
Create Feedback Loops:
Create channels, through which people can report information about the uses of AI or which citizens can express their concern, opinion, or experience in this sphere.
Use Educational Campaigns:
Publicize AI via awareness creation, a media communication tool, or an information exhibit that informs the general public.
Measure Trust Metrics:
To this end, public opinion polls should be carried out in order to know how the public views the application of AI so that the approach used in the application of AI can be modified where necessary.
Foster Open Dialogue with Citizens:
Therefore, communities should discuss the AI projects and address issues concerning it with intentions of creating awareness of the gains. Dedication to the process of open and honest dialogue creates the right level of trust between parties.
Promote Public Awareness Programs:
PPC advertisements should be conducted to help the public understand what AI is, what it is capable of doing and what it cannot do. This includes the participation of professionals in AI, to ensure that common language is used while explaining the impact of AI to society.
Share Real-World Case Studies:
Provide examples and use cases of technologies already being used by police forces around the world to solve real problems and increase safety.
Encourage Public Participation in AI Policy:
Let citizens have a say in decision making concerning the definition of AI related policies. This may include conducting research on citizens’ opinions through surveys, conducting workshops or engaging the public in a consultation process to ensure that the use of artificial intelligence is advocated in areas that are in line with the needs of the community.
Establish Community Advisory Boards:
Establish local advisory boards for the AI project with members of the population, so the developed solutions will correspond to the peculiarities of ethical and societal norms.
Address Concerns Promptly and Transparently:
Use reason to address or explain the public areas of concern about AI in an accountable way. Helping people understand and quickly solve their problems creates credibility with them.
Provide Clear Communication Channels:
Organize clear channels, which include call lines or online sites, through which citizens can call, give their opinions or contact the authority for information on the current AI projects.
Host Town Hall Meetings for Feedback:
Hold frequent meetings with the teams responsible for AI progress and future of policing plans and what difficulties they face in the process. Promote the people’s participation in the community and ensure that they give their opinions.
Build Partnerships with Local Organizations:
Engage the community groups, business organizations, and appropriate non-governmental organizations to ensure that solutions include the required artificial intelligence tools in the locally identified problem areas; thereby encouraging ownership of the program.
Highlight Positive Impact Stories of AI in Law Enforcement:
Post stories of how AI has impacted public safety, crime and justice, to create perceptions of AI as an instrument for the common good.
That is why public engagement is considered the most critical aspect of establishing confidence in the use of AI to support law enforcement functions.
Are We Prepared for Legal Challenges?
Adhering to the national and international standards helps to reduce the risks which are always existed in law enforcement’s sphere. That brings us to the question of how agencies stay compliant.
Follow Regulatory Frameworks:
Compliance with the regulations of AI in the United States and guidelines of AI systems in the global world.
Develop Internal Guidelines:
Propose a set of fundamental policies to explain how AI solutions must work under the existing laws.
Monitor Relevant Case Law:
Be up-to-date with the legal cases that impact the performance of AI systems.
Establish Liability Protocols:
Have clear guidelines on how to deal with cases of malconduct, abuse of data or the algorithms being wrong.
Collaborate with Policymakers:
Working with the legislators to ensure that the strategies formulated fit the new regulations in use of artificial intelligence.
Review Industry-Specific Legal Requirements:
Carry out research on the laws and regulations relevant to an industry of the application of AI in the respective industries. This helps meet addresses the requirement of certain sectors like healthcare, financial or education sectors.
Conduct Regular Legal Compliance Audits:
It is important to conduct annual checkups to ensure that all aspects of the business are in compliance with the AI-related laws, and to take action in case of legal risks.
Establish Legal Risk Management Protocols:
Develop methods for evaluating, controlling, and managing the legal implications of the use of AI, and intellectual property, liability, ethical issues, etc.
Collaborate with Legal Experts and Consultants:
Consult with legal experts on matters of AI law and ethics in order to have effective approaches to developing adequate laws and to identifying new issues.
Create AI-Specific Legal Frameworks:
Create internal policies and frameworks for the AI technologies unique to the organization and that address legal issues like algorithmic explanations.
Stay Updated on International AI Laws:
It is recommended to watch out for any shifts happens in the regulation of Artificial Intelligence across the world to maintain standards as set by global law in various regions.
Implement Data Protection and Privacy Standards:
Ensure data privacy acts like the GDPR or CCPA are followed by implementing strong privacy policies in the AI systems that process personal information.
Develop Procedures for Legal Dispute Resolution:
Put in place internal mechanisms for the handling of legal issues that would arise from the use of AI to avoid protraction and unfair treatment.
Train Legal Teams on AI Implications:
Conduct targeted educational sessions to improve legal departments’ awareness and knowledge of the ways that AI can influence compliance, IP, and regulatory issues.
Maintain Transparent Legal Documentation:
Maintain detailed documentation of AI processes, decision-making, and compliance initiatives to promote transparency and build trust with stakeholders.
My advice? The sooner you start legal preparations the better! In its essence, the concept of proactive governance helps reduce the incidences of operational disruption.
How Can We Protect Data Privacy?
Loss of data privacy compromises the capabilities of AI and the public’s confidence in the technology. It is particularly important for me as the head of the agency to guarantee highly effective privacy protocols in the agency.
Limit Data Collection:
This only means that there should be a restriction for the kind of information that is being shared and analyzed.
Upgrade Encryption Levels:
For personal or policy only data, apply the most advanced methods of data protection.
Apply Strict Access Policies:
Apply check to discourage the unauthorized employees or third parties from access.
Develop Data Retention Rules:
Appropriate goals and objectives need to be established on how long information will be retained and when it will be expunged.
Audit Regularly:
Perform assessments in order to make sure systems are compliant with such laws as GDPR or CCPA.
Draft Breach Protocols:
Make sure you have a fast and straightforward plan on what should be done once the breach of the affected data is realized.
Implement Data Masking Techniques:
Use data masking to translate sensitive information into forms of fake information hard to differentiate from the original data during testing or development but safe to use.
Monitor Data Access Logs:
It means that one should review or audit the access logs frequently in order to identify the suspicious activities or attempts at unauthorized access of ones data systems.
Conduct Privacy Impact Assessments:
Assess potential privacy threats that may affect new product development or information technology to meet and meet legal requirement before going live.
Encrypt Data at Rest and in Transit:
Employ the SSL to protect data from external threats, especially at the moment of its storage and during data transfer.
Adopt Privacy by Design Principles:
Design them into the overall system, a product or a process, therefore making privacy protection an inherent component of the system.
Provide Privacy Training for Employees:
Provide clear guidelines on how staff should and should not interact with personal and institutional information in order to reduce privacy risks and encourage all employees to take privacy seriously.
Establish Data Anonymization Practices:
Develop measures that would minimize the identifiable information in the data sets so that risk of re identification is minimal while the analysis is maximized safely.
Utilize Secure Data Storage Solutions:
Maintain data in systems that have extra security measures that include encryption of data, restricted access, and constantly monitored to guard against intruders.
Ensure Third-Party Data Compliance:
Ensure that any third-party vendors or partners understand the privacy regulation and the contractual conditions related to the data they receive.
Maintain Transparent Privacy Policies:
Inform users about your company’s data policies and privacy policies to ensure you have their investment and to meet legal obligations.
Ensure the protection of privacy to protect the system and the public image it will create.
Responsible AI Governance Can Lead Us Forward:
The use of AI in the Police force is evident, and can now lead to enhanced decision making and effective working. However, its use needs to be done so in ways that don’t compromise the principles of ethics, law, and public opinion.
Through strict vendor selection; including training programs; transparency; and, most importantly privacy and legal requirements in preventing mishaps, I am confident that the law enforcement agencies can properly leverage on AI.
Final Thoughts:
The use of AI in law enforcement is a challenging yet inevitable process which requires us all to be answerable for fairness, transparency and accountability in policing. On the following points: ethical concerns that need due consideration, public acceptance of AI and its implications, and the reinforcement of proper governance structures to ensure the rights’ protection, it is possible to reveal the approaches by which underlying opportunities of AI may be revealed while fulfilling the adequate protection of core rights. Together we have the change to determine how technology supports justice in an ethical and efficient manner.
What steps are you taking currently to meet these challenges? Which gaps in knowledge might be detrimental to AI oversight? Please feel free to write your thoughts or anything you would like to ask in the comment section down below because your opinion is as valuable.
-
Artificial Intelligence7 months ago
What is Artificial Intelligence? A Comprehensive Guide for Businesses and Enthusiasts
-
Artificial Intelligence4 months ago
How to Use Grok AI: A Complete Guide
-
Artificial Intelligence6 months ago
Unlocking the Power of Artificial Intelligence Tools
-
Artificial Intelligence6 months ago
What is DeepSeek? Revolutionizing AI with Cutting-Edge Solutions
-
Artificial Intelligence2 months ago
Meta’s AI Push: The Standalone Assistant App Set to Rival ChatGPT
-
Artificial Intelligence2 months ago
AI Technologies in Warehouse Automation:
-
Artificial Intelligence2 months ago
How Artificial Intelligence is Revolutionizing Logistics:
-
Artificial Intelligence6 months ago
What is Quantum Artificial Intelligence? How It Works and Why It Matters